Revolutionizing the software development landscape, this article delves into the symbiotic relationship between Artificial Intelligence (AI) and data engineering. Unraveling the impact of AI on data engineering processes, it explores key areas such as automated data cleaning, predictive data modeling, intelligent data integration, dynamic resource allocation, and the role of AI in handling Big Data challenges.
Harnessing the capabilities of Artificial Intelligence (AI) to elevate the performance of product engineers has become a pivotal endeavor in the fiercely competitive software domain. The pressure to deliver top-notch solutions promptly, avoiding delays and ensuring optimal quality, underscores the need for innovative approaches. Traditional methodologies sometimes fall short, necessitating the exploration of advanced strategies and the integration of AI tools to secure a competitive edge.
Accelerating Market Entry with AI Tools:
Our seasoned experts attest that the utilization of AI tools can expedite market entry by a staggering 20%. This results in a streamlined product launch cycle, reducing it to a mere 4 months compared to the 5 months required by conventional methods. The reclaimed time can be reinvested to enhance product quality or introduce additional features, providing a distinctive competitive advantage.
Understanding the Role of AI Tools:
Before delving further, it’s crucial to dispel the notion that AI tools replace human efforts or resolve intricate challenges independently. Instead, a more pragmatic approach is to view AI tools as aids that dissect challenges, refine issues, address them individually, and then amalgamate these polished solutions into a comprehensive answer.
Enhancing Engineering Productivity:
Incorporating AI into engineering workflows yields dividends in productivity. By automating routine tasks, AI liberates engineers to focus on the more intricate and creative aspects of product development. Tools like AWS CodeWhisperer and GitHub Copilot enhance code completion, potentially accelerating the coding process by 20-40%, depending on team expertise and technological context.
Improving Code Quality with AI:
AI plays a pivotal role in code refactoring, early bug identification, and vulnerability assessment, leading to improved code quality and reduced late-stage issues. AI-enhanced automated testing streamlines the testing process, resulting in more efficient deployments and a faster time-to-market.
Predictive Analysis in Product Development:
Leveraging AI-driven predictive analysis transforms the planning and execution phases of software development. By analyzing historical project data, AI tools accurately forecast project completion, timelines, potential roadblocks, and even anticipate potential system breakdowns.
Designing User-Centric Solutions:
AI’s insights into user behavior empower engineers to craft more user-centric solutions. Tools like Adobe’s Sensei and Dawoteil optimize designs based on user feedback, providing clear traceability of insights and intuitive navigation. Maze facilitates usability tests with AI-powered prompts and high-level analysis.
Continuous Learning and Refinement:
AI models continuously analyze and learn from the software development process, adapting to past mistakes, user feedback, and system performance to suggest areas of improvement. User feedback on generated results contributes to further model improvement.
Supporting Data-Driven Decision-Making:
AI analyzes vast amounts of data to provide actionable insights crucial for decision-making in software development. ChatGPT, for example, automates what-if and trend analyses, summarizes complex information, and aids in drafting governance documentation.
Augmented Security:
AI-powered security tools reduce the time spent on vulnerability patches, allowing engineers to focus on core product features. In cybersecurity, AI evolves from reactive to preemptive threat intelligence, offering real-time anomaly detection and response automation.
Increased Efficiency, Improved Accuracy, and Reduced Costs:
AI automates routine tasks, enhances accuracy, detects new malware variants, reduces false positives, and streamlines the handling of large data sets, ultimately reducing costs and improving overall efficiency.
Real-Time Threat Detection and Competitive Advantage:
AI processes data swiftly, enabling real-time threat detection and response, providing a competitive advantage of up to 40% acceleration in coding processes. Businesses adopting AI tools enhance workflow and gain a tangible lead in market share.
Conclusion:
AI tools emerge as transformative allies in software development, ensuring up to a 40% quicker time-to-market and reinforcing a company’s competitive edge. However, recognizing their limitations is crucial, especially in scenarios involving intricate logic, infrequently used components, or tasks demanding profound contextual understanding. While AI integration propels modern product engineering, discerning its applicability remains paramount to unlocking its full potential.
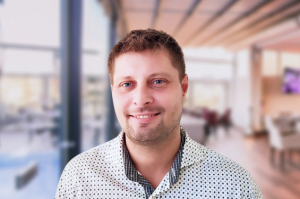
- Accelerating Market Entry with AI Tools:
- Understanding the Role of AI Tools:
- Enhancing Engineering Productivity:
- Improving Code Quality with AI:
- Predictive Analysis in Product Development:
- Designing User-Centric Solutions:
- Continuous Learning and Refinement:
- Supporting Data-Driven Decision-Making:
- Augmented Security:
- Increased Efficiency, Improved Accuracy, and Reduced Costs:
- Real-Time Threat Detection and Competitive Advantage:
- Conclusion:
Explore more topics
Talk to Our Talent Expert
